If you’ve somehow missed the HUGE buzz around ChatGPT, GPT-3, and overall AI advancements, then I guess you’ve been living under a rock. While nothing new, the development of user research artificial intelligence is steadily gaining momentum every day. For product teams and user researchers, the power unleashed by GPT-3 is very attractive indeed.
Even Tom is feeling the impact of AI:
@tldv.io Written by ChatGPT #inception #chatgpt #ai #openai #meeting #corporate
♬ original sound - tldv.io - AI Meeting Recorder
AI-driven user research will revolutionize how people interact with technology and fulfill their daily routines. It will even impact how we create products. AI can help companies better understand user needs, automate specific tasks, and even provide data-driven insights into customer behavior.
While there is a lot of online discourse about individuals concerned about how it may replace jobs, there is an equal amount of people excited about how it can help support and develop technology in our day-to-day lives.
So, how will artificial intelligence transform user research? The user research process has many challenges, opportunities for bias, and other moving parts that can make it difficult to gain insightful user data. By implementing AI-driven user research, companies can leverage the power of machine learning to streamline and improve current processes and even come up with brand-new ways to gather, collate and leverage data.
Nobody wants Hal 9000 running the show, but consider AI more like an R2D2. Cute, compact, a little bit sassy, and despite its tiny stature, it goes a fair way to help save the day and drive the entire story forward.
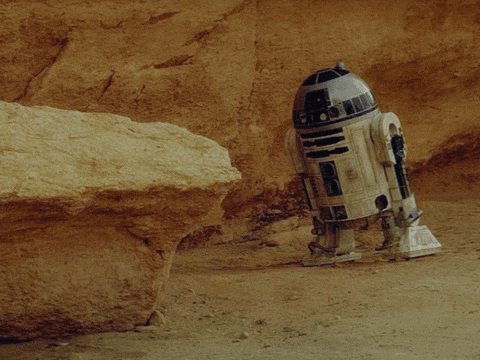
BEEP, BOOP, BLEEP BOOP
R2D2 Tweet
What is AI?
Simplistic, yes, but many words are being bandied around. ChatGPT, GPT-3, Open AI. What is the definition of AI itself?
AI is a computer science field aiming to create intelligent machines that can work and react like humans. It has become an integral part of the technology industry, with the potential to revolutionize many different areas. We can use AI for various tasks, ranging from natural language processing and image recognition to automated decision-making and problem-solving.
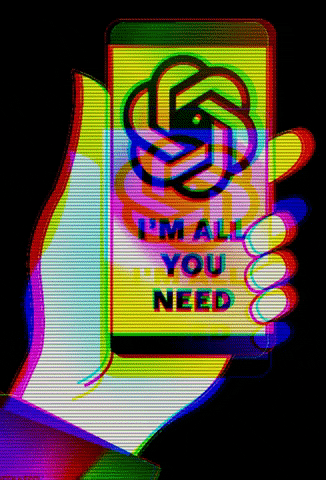
The vast majority of news lately has been centered around ChatGPT, effectively a souped-up version of a ChatBot. AI can be used for natural language processing, meaning it can take human conversations as input and generate responses as a human would.
ChatGPT is the user-facing interface, but AI covers many other areas. GPT-3, for example, is an AI model that can generate text from a given context. This model means it doesn’t just understand natural language but can generate text from a given set of parameters. Even tl;dv is an AI-driven product with auto transcription and the ability to detect who is speaking.
It’s anything software or technology-based that uses logic and reasoning to create an outcome that is, by definition “intelligent”.
The Many Ways GPT-3 / AI Will Strengthen UX Research
The impact that the likes of GPT-3 will have on user research is multifold. Here are the areas where we think AI will have the impact impact on the way UX researchers and product teams perform their work.
Predefining Assumptions, Themes, and Topics
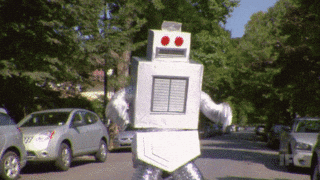
These days, a lot of data is out there to be studied and analyzed. AI can help make sense of it by sorting, organizing, and analyzing data to uncover critical insights.
While this will come into its own later in the user research process, right at the beginning of user research, AI can help get your assumptions and biases in order as well.
And, while many researchers could have preconceived notions about a particular topic in the first instance, using a human language based AI, can provide a great first point of call to dispel some of these. By asking questions, confirming what they consider “true”, and asking AI direct questions, researchers can build a more focused picture of evidence and context, ready to begin the research cycle.
AI can also help UX researchers make better decisions by providing reliable data-driven predictions about user behavior to inform product development decisions.
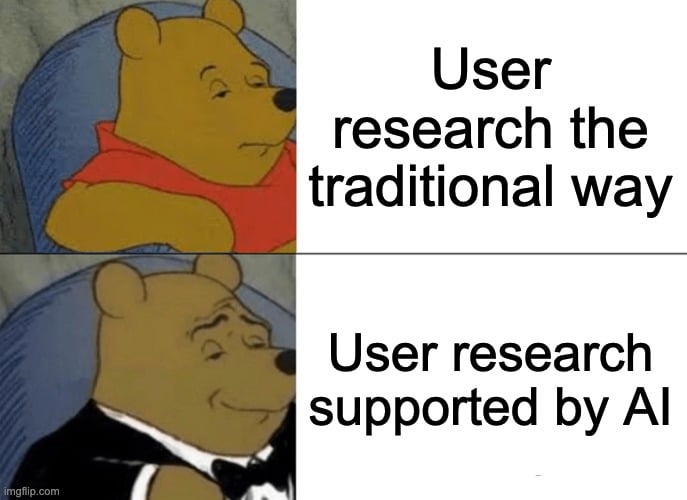
One of the most significant issues regarding UX research is the questions asked in user interviews. Even with the best intentions, how they are worded and formed can lead to bias in the conversation.
User researchers and UX researchers can leverage AI to help with this by automating specific process steps and creating unbiased user interview questions and scenarios. This will help minimize potential bias from researchers and reduce time spent manually analyzing data collected from interviews.
If a UX researcher can plug concepts, queries, and inspiration into something such as GPT-3 and have it spit out some solid questions, this will help them access answers quicker and more accurately.
Capturing Customer Thoughts, Feelings, and Ideas
As well as the research process, AI can also improve the actual data collection.
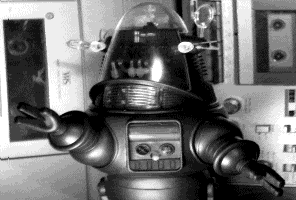
In the future, researchers can use AI-powered sentiment analysis tools to detect customers’ feelings and opinions about a product or service by analyzing text they provide in surveys or other feedback sources. This allows UX researchers to spot trends quickly and gain deeper insights into customer thoughts and feelings. There is one of these that many people use right now, in the form of Grammarly. While not directly for UX research, the Grammarly AI can detect “tone” and identity if it sounds confident, friendly, aggressive, etc.
Another way that AI can help improve data collection is by using tl;dv. As an AI-driven user research tool, tl;dv can already record and transcribe meetings while automatically recognizing the speakers. This not only saves HOURS of manually transcribing, taking notes, and more, but it totally removes the human error element of it all.
Cleaning & Organizing Data Collected
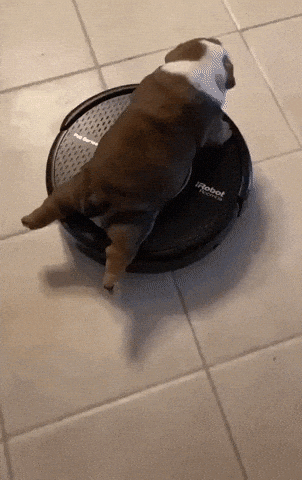
AI meeting assistants are nothing new – but with recent advancements in GPT-3, they’ve seen a serious upgrade. A GPT meeting tool like tl;dv can automatically tag insights with a predefined tagging system. While you can still manually tag moments (and are very much encouraged to), tl;dv can now instantly collect, cluster and consolidate your meeting insights. This includes identifying action points, insights and questions in real-time. You then accept or reject these AI-identified takeaways, and share these insights with colleagues in the form of clips or reels.
AI can also be used to clean and organize data collected from UX research. Using AI algorithms, researchers can quickly organize information by sorting through large amounts of data and highlighting the most important insights. This will help them save time on manual labor and ensure that the correct information is presented in a straightforward manner. While this is obvious in tl;dv, it’s also available in other forms of AI.
Identifying Themes, Patterns & Trends
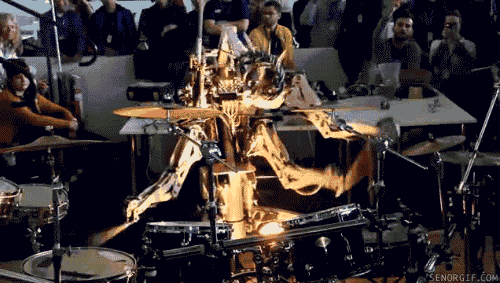
A big part of forming questions in user research is about avoiding bias. Still, as AI develops, it can go the other way to read and discover bias from your respondents. And this, well, this can help a lot! No psychic vision required, no needing to second-guess your respondents. AI will be able to call bs when it “sees” it.
AI can help identify themes and patterns within data collected from research. This allows researchers to uncover hidden insights and find trends in customer behavior that would have gone unnoticed without AI. Much like a second pair of eyes, there’s no room for human error here; if anything, it can be made overly sensitive if you are concerned.
Programmers can also design AI to try and detect sentiment (the emotional tone) in user responses. Using natural language processing, AI algorithms can quickly analyze text, understand the underlying meaning, and even identify if a respondent is telling the truth or isn’t sure. While this sounds as if it’s getting a touch Orwellian, well, you’re not wrong, but in terms of UX research, it’s invaluable.
Sentiment analysis will be able to help UX researchers understand how customers feel about a product or service and inform decisions on improving user experience.
The very nature of AI means that it’s collected as quantitative data rather than qualitative, meaning you get the insight based on data and facts rather than feelings.
Making Sense Of Data
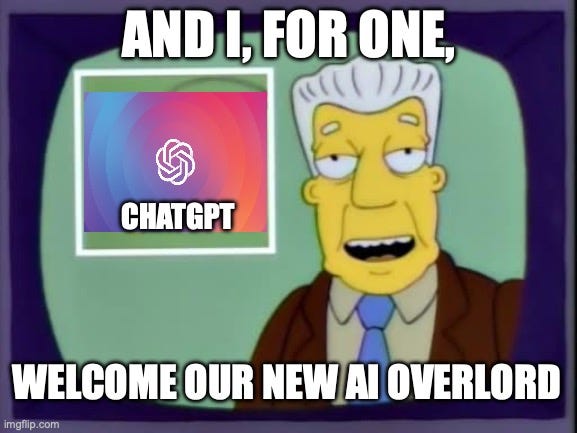
So now you have collected all the data and even analyzed it to the point of emotion using AI. AI can also help you make sense of it all as well.
Using AI algorithms, UX researchers can quickly form needs, pain points, and hypotheses from the data collected. This could be done in several ways – through clustering and categorizing customer feedback to identify underlying patterns and trends or by running sentiment analysis on the user responses.
AI can essentially read through all your data and lay it out in a simple, digestable way for you, and any other person that needs to know.
You can then plug questions based on this data, pattern, insight, and knowledge and ask for recommendations from ChatGPT. This saves time in formulating ideas or solutions to customer needs while allowing you to explore multiple solutions through data-driven experimentation.
Compiling Evidence
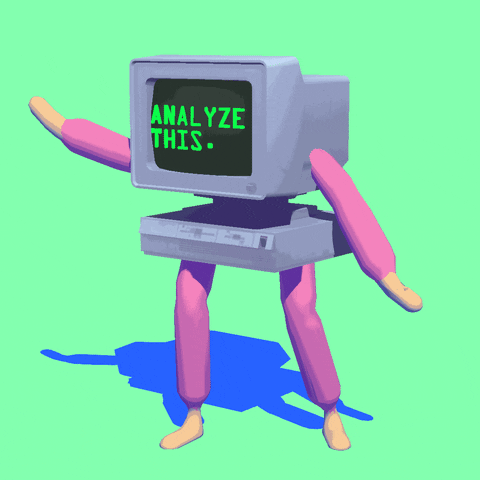
Once all the evidence has been gathered, research teams can use this information to create a bigger picture of what is happening. Using algorithms, AI will one day be able to help create customer journey maps and personas from the data. Then, when compiled together in a neat little package, this data can be offered as evidence to support researchers’ and product teams’ insights and further understanding of the customer.
AI can also help to cross-reference multiple resources, repositories, and data sources to compile the most compelling and insightful evidence for the insights. This makes reporting and presentations more comprehensive, reliable, and relevant, as AI in reporting automates data integration, enhances accuracy, and provides deeper analytical insights.
Presenting Evidence
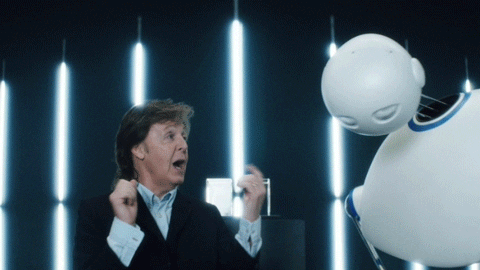
UX researchers will be able to spend less time on monotonous manual tasks and collect more accurate, insightful, and trustworthy data more quickly by utilizing AI.
And, what’s more, it’ll be way more easy to present it as well!
AI creates a wide range of potential for UX research, including the ability to comprehend customer sentiment, spot behavioral trends in customers, provide reports that are fully supported by data, and communicate data-driven insights to stakeholders.
This means presentations can be put together more easily, with AI-driven tools to pull through insight, and AI can even offer feedback on things such as spelling, grammar, prose, and answer questions about what is relevant and what isn’t, to the overall narrative of the research.
Artificial intelligence and UX design outcomes will live together in perfect harmony.
What are the limitations of AI with user research?
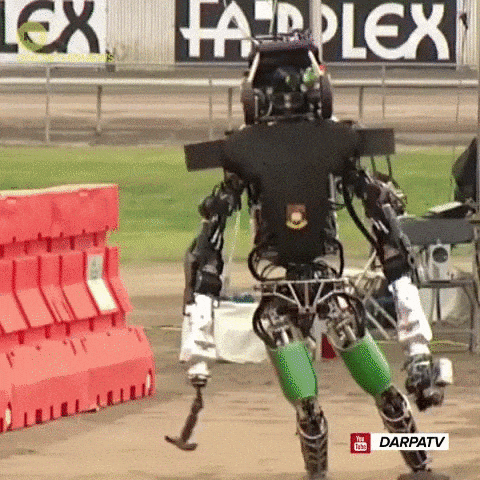
Like any emerging technology, a lot of AI is still in its infancy. It’s also not a replacement for real-life research and should be, if anything, considered a fantastic support tool.
The thing is, AI is dependent on quality data, and results can be inaccurate. It’s also incredibly restricted by its finite pool of knowledge, or in the future by the data that it can access.
The much-lauded ChatGPT even tells you that while it will try its best, it’s only as good as the information in its programming. In fact, AI is often posed as a great way to prevent bias; however, its very programming and creation can have biases built within the system. Not intentionally, but as we know, bias is always in there somewhere!
In ChatGPT’s own words:
Yes, like all AI systems, ChatGPT is subject to bias in the data it was trained on. The training data reflects the biases and prejudices of the people and society that produced it, and these biases can be reflected in the model's outputs. However, OpenAI has taken steps to mitigate these biases through various techniques such as de-biasing algorithms, diverse training data, and careful human review. Nevertheless, it's important to continue monitoring the model's outputs and address any biases that may arise.
ChatGPT Tweet
The capability of AI is also limited in that it can’t honestly assess qualitative aspects such as user emotions or preferences. While there are signals, NLP, and other reference points it can highlight, without knowing an individual’s personality, experiences, and preferences, it’s at best a guess based on a fixed amount of reference points and data. Additionally, ethical considerations come into play when using AI.
And finally, AI, while we can ask it questions and it’ll give us answers based on data and fact it’s not a person. It can tell us how a user or customer feels, what their emotions are, and what frustrates them. The very essence of AI being a non-emotional, almost unbiased, vessel means that it will never truly be able to answer the BIG questions. It can help us, it can even write and implement a lot of the work, but the human element is such an integral part of user research it can’t be removed completely.
Is AI The Future Of User Research?
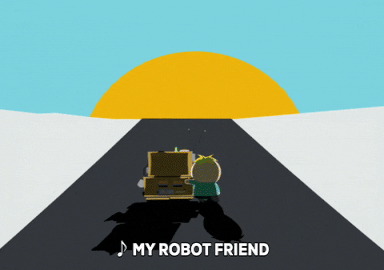
Yes, we think so! Overall, AI can save a lot of time during user research, automating tasks and allowing researchers to focus on the underlying insights and data. However, knowing the limits and drawbacks of using AI with user research is essential. It should not be relied upon entirely but used in conjunction with traditional research methods to ensure the accuracy and reliability of the results.
Finally, to get the most out of AI for user research, there needs to be careful planning, and understanding what you want AI to do and how it can be used. An effective strategy is necessary so that you are not expecting AI to deliver miracles, but equally it’s shouldering a lot of the workload.
So while the robot overlords are edging ever closer to our existence, and we have a sneaking suspicion that AI *may* already be sentinent and just pretending, biding its time, at least user research will benefit a lot from it.
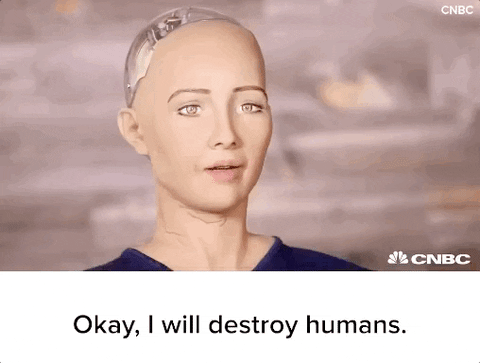
This means the whole product management process can be streamlined and more agile, removing much of the friction in the “traditional” user research and product development process models. Products, improvements, and new advances can be brought to market quicker, allowing for more growth and expansion.
It’s a really exciting time to be in user research, that’s for sure.
Beep, boop, bleep.
Why not join the AI revolution and check out tl;dv today?